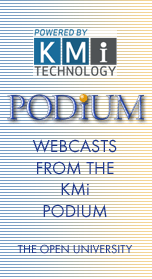
|
 |
This event took place on 7th September 2022 at 1:00pm (12:00 GMT)
Knowledge Media Institute, Berrill Building, The Open University, Milton Keynes, United Kingdom, MK7 6AA
Evaluation of machine learning models often involves a two stage comparison of model predictions to a human annotated "gold standard" - yielding a metric score such as accuracy or correlation - and then a comparison of those metric scores between a baseline model and some proposed improvement to it. These comparisons are used to e.g. establish new ``state-of-the-art'' results via benchmarks in the literature, or in practice to evaluate whether an engineering or data change made things better or worse. For the past decade of advances in AI, a mechanism to measure the confidence of these two-stage evaluations have eluded the community, and therefore we have largely failed to provide a measure of confidence on the comparative performance of the machines, even if statistical guarantees are provided for the individual stages. We propose that this ranking of machines should be grounded in a notion of statistical significance, and that grounding must be robust in the face of the multiple stages of comparison. In this work, we explored the production of p-value confidence scores for the models' comparative performance, by testing a null hypothesis that the machine predictions being compared are drawn from the same distribution. We then developed an approach to producing two-sided horizontal and vertical variance that allows us to test this null hypothesis and produce a p-value for the comparison of two sets of machine scores (e.g. proposed vs. baseline). In order to evaluate the p-values we produce, we developed a simulator that allows us to experiment with different metrics, sampling methods, and comparative distributions. Our initial results provide insight into which sampling methods and metrics provide the most accurate p-value for machine comparisons. |
The webcast was open to 300 users
|