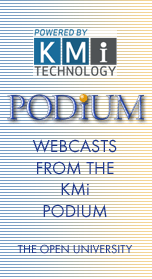
|
 |
This event took place on 12th October 2022 at 11:30am (10:30 GMT)
Knowledge Media Institute, Berrill Building, The Open University, Milton Keynes, United Kingdom, MK7 6AA
Demand for fairer machine learning models is rapidly growing due to their increasing use in many decision-making processes. Several methods have been developed to detect and mitigate the bias of these models. One common approach for addressing such bias is simply dropping the sensitive attribute from the training data (e.g. gender). Such an approach is limited by focusing on the fairness of the training process rather than on the output model. This kind of simplistic approaches overlook the fact that sensitive attributes can be indirectly represented by other attributes in the data (e.g. maternity leave taken). However, there is currently little research aiming at understanding how covariance in data can contribute to the propagation of bias in machine learning models. In this seminar, we use feature selection techniques and statistical tests to study the covariance of these attributes and show how this covariance can help explain model bias in credit risk data. We further demonstrate how fairness can be significantly improved by eliminating the related attributes and the subsequent impact on model accuracy. |
The webcast was open to 300 users
|