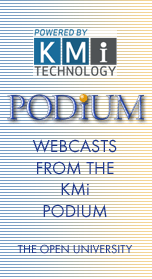
|
 |
This event took place on 16th July 2014 at 11:30am (10:30 GMT)
Knowledge Media Institute, Berrill Building, The Open University, Milton Keynes, United Kingdom, MK7 6AA
Prashant Aparajeya will present the research project "Point-based medialness for shape representation, recognition and movement computing" that he is conducting at Computer Vision group, Goldsmiths, University of London. In this work (in support of his PhD supervisor Prof. Frederic Fol Leymarie), they have introduced the idea of using a perception-based medial point description (medialness) of a deforming or moving 2D object as a framework for a part-based shape representation, which can then be efficiently used in dynamic pattern recognition and shape matching tasks. The first step is one of fuzzy medialness measurements of 2D segmented objects from intensity images which emphasises shape information characteristic of an object’s parts (such as the main concavities and folds along a contour). They distinguish interior from exterior shape description. Interior medialness is used to characterise deformations from straightness, corners and necks, while exterior medialness identifies the main concavities and inlands. In the second step they define an interior dominant point as a well localised peak value in medialness representation; this is extracted using a top-hat transform from mathematical morphology. An exterior dominant point is evaluated by identifying a region of concavity sub-tended by a minimum angular support. Furthermore, convex point are extracted from the shape to find the blob like structure in the shape. Their evaluated feature points are invariant to shape movement up to a greater extent, where the articulation in moving objects are characterised by exterior dominant points. In the third step, an inelastic shape matching algorithm is designed that finds the most relevant target from a database of templates by comparing the dominant feature points in a scale, rotation and translation invariant way (inspired by the SIFT method). The performance of this method has been tested on several databases. The robustness of the algorithm is further tested by perturbing the data-set at several scales. Also, they propose that their evaluated feature points are sufficiently representative, as a basis for shape characterisation, to address many of the goals of movement computing. Along with this, their exploration includes the use of the main psychologically-motivated point descriptors of a shape in studying and characterising different gestures, including those performed by a human artist when performing graffiti (street) art. One of their goal is to be able to develop learning by example shape-based gestures first to duplicate an artist's expertise via robotic platforms and later engage in human-robot collaborations in artistic creativity. |
The webcast was open to 1000 users
|