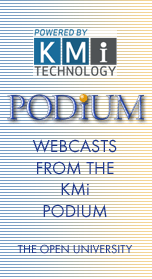
|
 |
Privacy-Preserving Decentralised Learning Analytics: Differential Privacy
Audrey Ekuban
This event took place on 25th January 2023 at 11:30am (11:30 GMT)
Knowledge Media Institute, Berrill Building, The Open University, Milton Keynes, United Kingdom, MK7 6AA
Predictive Learning Analytics (PLA) is a subfield of Learning Analytics that uses Student Activity Data, such as Assessment Scores and Trace Data, to create predictive Machine Learning models. These models are then used to provide insights to course tutors, who can then offer timely assistance to students at risk of not successfully completing a course. Despite its usefulness, there are some issues with the typically centralised approach to PLA. These include concerns about ethics, privacy and security associated with big data, and difficulties with application to a student's lifelong learning. The main hypothesis of my PhD is that these concerns could be addressed by adopting a decentralised approach to Learning Analytics. With the growing sophistication of methods to re-identify individuals from anonymous datasets, an individual’s privacy cannot be guaranteed by using pseudonyms or k-anonymity. Differential Privacy (DP), a technique that adds statistical noise to data, is increasingly being used to protect the privacy of individuals in a dataset. However, it is known that there is a trade-off between privacy and the accuracy of any data analysis. By the addition of random noise to aggregated data, Global DP aims to protect an individual's information from attacks that target aggregated data. It can be used alongside Federated Learning, a technique that enables a Machine Learning model to be trained on decentralised devices, without data leaving the devices. In this case, the weights of a Neural Network would represent the aggregated data. Local DP allows individuals to add random noise to their data and, hence, provides plausible deniability. In this talk, I will present ongoing work to investigate the privacy-accuracy trade-off in the use of DP in Decentralised Learning Analytics. |
The webcast was open to 300 users
|