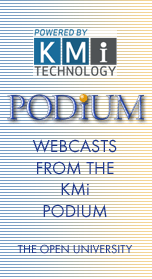 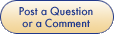
|
 |
Substituting Negated Features with Antonyms for Sentiment Classification
Shahzad Khan
This event took place on 8th March 2006 at 9:00am (09:00 GMT)
Knowledge Media Institute, Berrill Building, The Open University, Milton Keynes, United Kingdom, MK7 6AA
Sentiment classification (SC) is a novel extension of the text classification framework. We demonstrate that linguistic negation analyses significantly increases the accuracy of SC by introducing useful new features to the supervised machine learning process and extend this research by employing knowledge of antonymy to reduce the new features introduced by the negation analysis. We hypothesize that the antonyms substitution enhancement should improve accuracy by encoding in the classification features the linguistic relationship that exists between negated terms and their antonyms. Our hypothesis is premised on the conjecture that negated features and their antonyms are equivalent, as both are used to describe a term?s ?opposite? concept. We discovered and overcame issues related to the weak coverage of antonym lists and the polysemous nature of the negated words by employing both word-sense-disambiguation and corpus based antonyms extraction methods. As negation and antonymy are both fundamental lexical-syntactic relations, these methods can aid a variety of supervised machine learning approaches to natural language and information processing.
Download PowerPoint presentation (44kb ZIP file) |
Return to the event page |
Click here to submit a question or comment
The webcast was open to 50 users
|
Click below to play the event (28 minutes) |
|
|