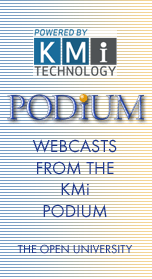
|
 |
Building Trustworthy AI: Uncertainty Quantification and Failure Detection in Large Vision-Language Models
Shuang Ao
This event took place on 28th May 2024 at 11:30am (10:30 GMT)
Knowledge Media Institute, Berrill Building, The Open University, Milton Keynes, United Kingdom, MK7 6AA
Although AI systems, especially LLMs, have been applied in various fields and achieved impressive performance, their safety and reliability are still a big concern. It is especially important for safety-critical tasks. One shared characteristic of these critical tasks is their risk sensitivity, where small mistakes can cause big consequences and even endanger life. Specifically, the current AI algorithms are unable to identify common causes for failure detection, as well as wrong predictions and OOD samples in inference. Furthermore, additional techniques are required to quantify the quality of predictions. All these contribute to inaccurate uncertainty quantification, which lowers trust in predictions. Hence obtaining accurate model uncertainty quantification and its further improvement are challenging. As vision and language are the most typical data types and have many open-source benchmark datasets, my work focuses on vision-language data processing for tasks like classification, image captioning, and vision question answering. My work aims to build a safeguard by developing techniques to ensure accurate model uncertainty for safety-critical tasks. |
The webcast was open to 300 users
|