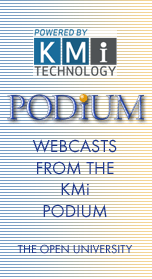
|
 |
Learning Conditional Random Fields from Unaligned Data for Natural Language Understanding
Dr. Deyu Zhou
This event took place on 28th October 2011 at 11:30am (10:30 GMT)
Knowledge Media Institute, Berrill Building, The Open University, Milton Keynes, United Kingdom, MK7 6AA
One of the key tasks in natural language understanding is semantic parsing which maps natural language sentences to complete formal meaning representations. Rule-based approaches are typically domain-specific and often fragile. Statistical approaches are able to accommodate the variations found in real data and hence can in principle be more robust. However, statistical approaches need fully annotated data for training the models. A learning approach to train conditional random fields from unaligned data for natural language understanding is proposed and discussed. The learning approach resembles the expectation maximization algorithm. It has two advantages, one is that only abstract annotations are needed instead of fully word-level annotations, and the other is that the proposed learning framework can be easily extended for training other discriminative models, such as support vector machines, from abstract annotations. The proposed approach has been tested on the DARPA Communicator Data. Experimental results show that it outperforms the hidden vector state (HVS) model, a modified hidden Markov model also trained on abstract annotations. |
The webcast was open to 100 users
|
Click below to play the event (38 minutes) |
|
|